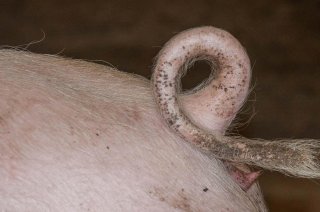
Project
Creating Resilience in Pigs Through Artificial Intelligence (CuRly Pig TAIL)
With current knowledge and practices tail biting seems hard to fully prevent. Tail biting is the result of reduced health or wellbeing of pigs, with a number of risk factors involved. An early warning system is not yet available.
The project Creating Resilience in Pigs Through Artificial Intelligence (CuRly Pig TAIL) aims to overcome this gap by developing an advanced non-invasive monitoring system for resilience in pigs. Intact curly tails are a flag of high resilience. Being able to raise pigs with intact tails is regarded as a significant indicator of a healthy, well managed and resilient pig and pig husbandry system.
Rendering meaningful signs of decreasing resilience
Single monitoring systems of external signals already exist. However, a system registering and interpreting individual and group behaviour does not. Combining relevant signals from the animal as well as its group and the surroundings of the animal to render meaningful signs of decreasing resilience has not been developed yet.
By using behavioural knowledge, computer vision and deep learning techniques, signals can be gathered and interpreted in an automated manner. Health problems, as well as disruptions in climate, feed and mental status (i.e. boredom) of pigs all lead to a decreased resilience and a higher exploratory need, often resulting in tail biting. Currently, almost all piglets are routinely tail docked at a young age to prevent this symptom of decreased resilience, but this procedure is subject to ethical and societal debate.
Deep learning uses machine-learning algorithms that can learn to detect patterns in the sensor data, which can be used for classification, partitioning and detection.
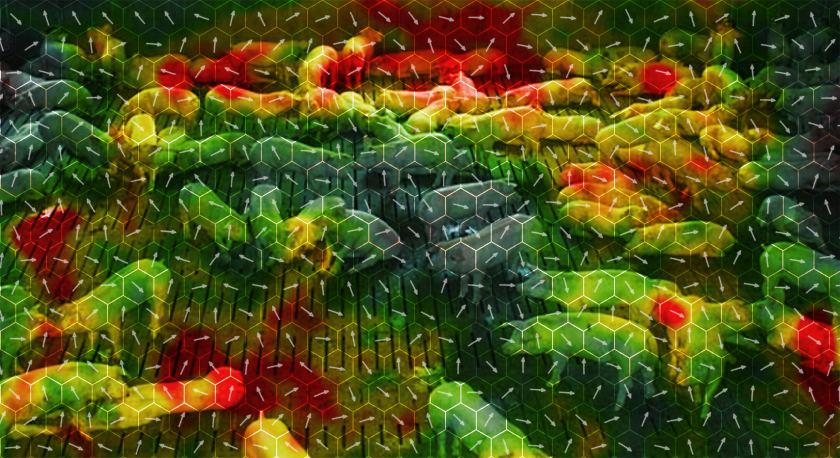
Pig’s tail posture and intactness crucial indicators
The aim of this research is to integrate interdisciplinary and transdisciplinary knowledge regarding pigs (welfare, health, physiology and ethology), computer vision and machine deep learning to develop an automated monitoring system which can detect multiple disturbances in the pig and the husbandry system (e.g. changes in tail posture, skin colour, flow patterns, climate, etc. ) which are known to endanger the resilience of individual pigs and groups of pigs. In this way, early interventions to restore resilience can be made, thereby improving pig welfare and health.
Our main indicator for resilience is the pig’s tail posture and intactness, but through our inter- and transdisciplinary approach also new indicators of (loss of) resilience may be found. By using technical aids 24 hours a day, the detection of a change in the tail can be made earlier than a pig farmer currently is able to, thereby enabling the possibility of early intervention to take preventive measures.
Pig steers technology
In our approach, the pig is the focus point and technology will be developed monitoring the needs and welfare of the pig. This animal and technology based approach will be integrated with the craftsmanship of the farmer and act as a supporting system. By communicating integrated signals to the farmer the resilience of the pigs can be guarded and enhanced when necessary and an improvement in the economic return can be achieved. The system can also be used to improve the communication and transparency between the different partners in the supply chain.
Pig’s tail posture and intactness crucial indicators
The aim of this research is to integrate interdisciplinary and transdisciplinary knowledge regarding pigs (welfare, health, physiology and ethology), computer vision and machine deep learning to develop an automated monitoring system which can detect multiple disturbances in the pig and the husbandry system (e.g. changes in tail posture, skin colour, flow patterns, climate, etc. ) which are known to endanger the resilience of individual pigs and groups of pigs. In this way, early interventions to restore resilience can be made, thereby improving pig welfare and health.
Our main indicator for resilience is the pig’s tail posture and intactness, but through our inter- and transdisciplinary approach also new indicators of (loss of) resilience may be found. By using technical aids 24 hours a day, the detection of a change in the tail can be made earlier than a pig farmer currently is able to, thereby enabling the possibility of early intervention to take preventive measures.

Pig steers technology
In our approach, the pig is the focus point and technology will be developed monitoring the needs and welfare of the pig. This animal and technology based approach will be integrated with the craftsmanship of the farmer and act as a supporting system. By communicating integrated signals to the farmer the resilience of the pigs can be guarded and enhanced when necessary and an improvement in the economic return can be achieved. The system can also be used to improve the communication and transparency between the different partners in the supply chain.
Economic, academic and social impact perspectives
Monitoring health and welfare of animals is a highly demanding task for the farmer, with regard to time and complexity. Round the clock automated monitoring support will enable early interventions, and lead to less costs and more revenues for the farmer. Diseases and tail biting in pigs cause a significant economic loss for farmers (€ 8 million per year for tail biting in docked pigs), but also lead to use of antibiotics, which is cause for concern. CuRly Pig TAIL will contribute to control the risk of disease and tail biting, thereby improving the image and profitability of the pig sector.
The academic challenge is to integrate expert knowledge (from institutes within Wageningen University & Research), deep learning, computer vision systems and system design to boost innovation with development and application of new technologies. Innovative discoveries could include new subtle pig signals that enable early detection of decreased resilience.
Tail docking is a procedure that has raised societal concern. Besides discussion regarding the integrity of the animal, intact and undamaged tails are seen as an important indicator of a resilient and healthy system. Healthy pigs with high welfare and an intact curly tail will improve the general public’s perception of the pig sector, which is currently very low.
Methodological framework
The pig provides a huge amount of information for its resilience status by its behaviour and appearance (e.g. lying, eating, skin colour, eye colour, hair coat and tail-posture). By automated (non-invasive) monitoring of signals of the individual animal (e.g. tail posture, wounds, skin colour, water and feed consumption) and flow patterns of groups of animals, we believe it is possible to identify (early) signs of decreasing resilience that can ultimately result in disease and undesired damaging behaviour.
Processing collected signals from the pig and its environment with a combination of computer vision and pig knowledge and analysing them with deep learning techniques renders meaningful signals of decreasing resilience to the farmer. By combining deep learning with optical-flow approaches that identify movements in the image, abnormal dynamic behaviour of the herd can be detected.
Approach: group + individual = health status
We propose to develop an automatic system that supports the farmer with 24/7 monitoring of the herd, providing an early warning of potential behavioural problems and information on the health status of the individual pigs. The proposed method consists of three parts.
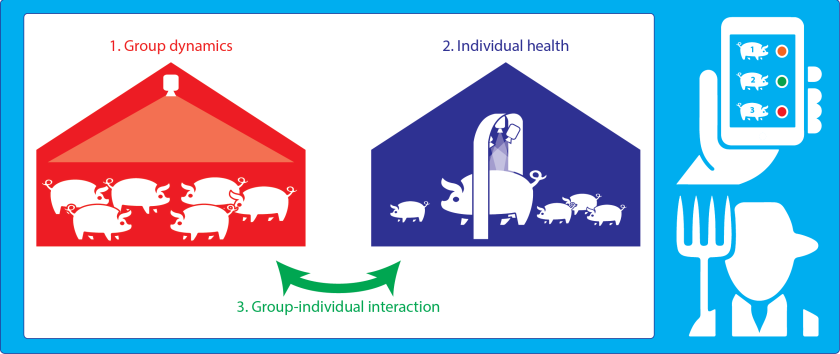
Part 1: group dynamics using optical flow
Group dynamics can be monitored by the flow of and interaction among animals (see figure). The flow encompasses the position and motion of the animals. Health and welfare issues associated with a change in group dynamics could be, e.g., infectious diseases, tail biting, aggression and boredom. We will investigate the relation between group dynamics and tail biting as a measure for resilience. A coarse analysis of optical flow has been shown to be predictive for animal welfare in, for instance, detection of African swine fever in pigs and several welfare measures in poultry.
We will describe the group dynamics using optical flow extracted from the images of overhead cameras. Optical flow describes the local motion in the image at a given time point. By registering the flow vectors over time, a spatial-temporal representation can be modelled probabilistically. Such a model gives the probability for motion in a particular direction at a particular point in space and time. During normal behaviour, the model will reveal the standard motion patterns of the herd. If at some point the motion pattern is classified by the model as unlikely, there is an indication that the behaviour of the herd is changing and the farmer can be warned.
Part 2: inspection of individual health
Body language and behaviour provides relevant information concerning the health and welfare of a pig. Signals are for example skin colour and temperature, eye colour, injuries (incl. tail wounds), respiration (rhythm, quality, coughing) and eating and drinking behaviour (e.g. number of meals, water consumption). Problems which can be detected by careful observation of the pig are for example diseases, decreased growth performance, welfare status (pain, stress, negative emotions), respiratory and gut problems.
To allow health inspection, we need to monitor the individual pig in great detail. We therefore envision a system equipped with multiple cameras and controlled illumination that functions as a gate to the feeding area, so that pigs pass the system multiple times a day. With this setup, a high-resolution 3D colour reconstruction of the animal can be determined, data that can be used for thorough inspection of the health status of the pig. We will use a subset of deep-learning methods, convolutional neural networks, to detect different body parts (e.g., tail, skin, eyes and ears). Using the same methods, the health status of the body parts can be determined. Positive indicators of health are, for instance a curly tail, clean skin and the absence of wounds. Additionally, volume information can be extracted from the 3D reconstruction to determine the growth of the animal. The networks will be trained on a set of data with healthy and unhealthy pigs, which are annotated by an expert in the field on animal welfare. The trained network will then be able to determine the health status for all pigs that enter the gate similarly to how the expert will judge it. By combining this with an RFID tag, health of each animal can be tracked and traced over time. Deviations from normal and healthy can be signalled to the farmer.
Part 3: Combining group dynamics and health of the individual pigs
Consulted literature
Anonymous, 2016. Sensoren kunnen gezondheid en welzijn varkens monitoren. VILT, België. http://www.vilt.be/sensoren-kunnen-gezondheid-en-welzijn-varkens-monitoren
Boumans, .I.J.M.M., E.A.M. Bokkers, G.J. Hofstede, I.J.M. de Boer. 2015. Understanding feeding patterns in growing pigs by modelling growth and motivation. Applied Animal Behaviour Science 171: 69-80. http://dx.doi.org/10.1016/j.applanim.2015.08.013
Boumans, I.J.M.M., G.J. Hofstede, J.E. Bolhuis, I.J.M. de Boer, E.A.M. Bokkers. 2016. Agent-based modelling in applied ethology: An exploratory case study of behavioural dynamics in tail biting in pigs. Applied Animal Behaviour Science 183: 10-18. http://dx.doi.org/10.1016/j.applanim.2016.07.011
Brunberg, E.I., T.B. Rodenburg, L. Rydhmer, J.B. Kjaer, P. Jensen, L.J. Keeling. 2016. Omnivores going astray: a review and new synthesis of abnormal behaviour in pigs and laying hens. Frontiers in Veterinary Science 3:57. http://dx.doi.org/10.3389/fvets.2016.00057
Cornou, C., A.R. Kristensen. 2013. Use of information from monitoring and decision support systems in pig production: collection, applications and expected benefits. Livestock Science 157: 552-567. http://dx.doi.org/10.1016/j.livsci.2013.07.016
Dawkins, M.A., R. Cain, S. J. Roberts. 2012. Optical flow, flock behaviour and chicken welfare. Animal Behaviour 84(1): 219–223. http://dx.doi.org/10.1016/j.anbehav.2012.04.036
Golbach, F., Kootstra, G., Damjanovic, S., Otten, G. and van de Zedde, R. 2016. Validation of plant part measurements using a 3D reconstruction method suitable for high-throughput seedling phenotyping. Machine Vision and Applications, 27(5): 663-680. http://dx.doi.org/10.1007/s00138-015-0727-5
Halachmi, I., A. Schlageter Tello, A. Peña Fernández, T. van Hertem, V. Sibony, S. Weyl-Feinstein, A. Verbrugge, M. Bonneau, R. Neilson. 2015. Discussion: PLF for automatic detection of animal health – poultry and pigs. Precision livestock farming applications: Making sense of sensors to support farm management; Ilan Halachmi Ed., 225-228. DOI: http://dx.doi.org/10.3920/978-90-8686-815-5_5.7
- He, K., Zhang, X., Ren, S., and Sun, J. Deep Residual Learning for Image Recognition. In: Computer Vision and Pattern Recognition (CVPR). 2016.
Hemeryck, M., D. Berckmans. 2015. Pig cough monitoring in the EU-PLF project: first results. Precision livestock farming applications: Making sense of sensors to support farm management; Ilan Halachmi Ed., 199-208. DOI: http://dx.doi.org/10.3920/978-90-8686-815-5_5.4
Kratz, L., & Nishino, K. 2009. Anomaly detection in extremely crowded scenes using spatio-temporal motion pattern models. In: IEEE Conference on Computer Vision and Pattern Recognition, 2009.
- Mahadevan, V., Li, W., Bhalodia, V., and Vasconcelos, N. Anomaly detection in crowded scenes. In: Computer Vision and Pattern Recognition (CVPR), 2010 IEEE Conference on. IEEE, 2010.
Martínez-Avilés, M., E. Fernández-Carrión, J. M. López García-Baones, J. M. Sánchez-Vizcaíno. 2017. Early Detection of Infection in Pigs through an Online Monitoring System. Transboundary and Emerging Diseases 64(2): 364-373.
Onwezen, M.C., M.J. Reinders and H.M. Snoek. 2016. Agrifoodmonitor 2016 - Hoe burgers de Agri & Food sector waarderen. Wageningen Economic Research, Wageningen. http://edepot.wur.nl/398441
Ramaekers, P.J.L., J.H. Huiskes, P.C. Vesseur, G.P. Binnendijk, H.M. Vermeer. 1996. Signaleren van afwijkingen in het eet- en drinkgedrag bij vleesvarkens. Proefverslag P1.142, Praktijkonderzoek Varkenshouderij, Rosmalen.
- Ren, S., He, K., Girshick, R., and Sun, J., Faster R-CNN: Towards Real-Time Object Detection with Region Proposal Networks. IEEE Transactions on Pattern Analysis and Machine Intelligence, 2016. 39(6): p. 1137-1149.
- Ryan, D., Denman, S., Fookes, C., and Sridharan, S. Textures of optical flow for real-time anomaly detection in crowds. In Advanced Video and Signal-Based Surveillance (AVSS), 2011.
- Shelhamer, E., Long, J., and Darrell, T., Fully Convolutional Networks for Semantic Segmentation. IEEE Transactions on Pattern Analysis and Machine Intelligence 2016. 39(4): p. 640-651.
Stern, U. R. He, C-H. Yang. 2015. Analyzing animal behavior via classifying each video frame using convolutional neural networks. Scientific Reports 5. http://dx.doi.org/10.1038/srep14351
Tsogkas, S., I. Kokkinos, G. Papandreou, A. Vedaldi. 2015. Semantic part segmentation with deep learning. https://arxiv.org/abs/1505.02438v2
- Turner, S. P., Nath, M., Horgan, G. W., Edwards, S. A. 2013. Measuring chronic social tension in groups of growing pigs using inter-individual distances. Applied Animal Behaviour Science 146: 26–36.
Ursinus, W.W. 2014. A tale too long for a tail too short? Identification of characteristics in pigs related to tail biting and other oral manipulations directed at conspecifics. Thesis. Wageningen University. http://edepot.wur.nl/314089.
Vandermeulen, J., C. Bahr, E. Tullo, I. Fontana, S. Ott, M. Kashiha, M. Guarino, C.P.H. Moons, F.A.M. Tuyttens, T. A. Niewold, D. Berckmans. 2015. Discerning pig screams in production environments. PlosOne, http://dx.doi.org/10.1371/journal.pone.0123111
Van der Peet, G.F.V. 2017. Staartbijten voorkomen bij varkens. http://www.wur.nl/nl/show/Staartbijten-voorkomen-bij-varkens.htm
Van Dixhoorn, I.D.E., I. Reimert, J. Middelkoop, J.E. Bolhuis, H.J. Wisselink, P.W.G. Groot Koerkamp, B. Kemp, N. Stockhofe-Zurwieden. 2016. Enriched Housing Reduces Disease Susceptibility to Co-Infection with Porcine Reproductive and Respiratory Virus (PRRSV) and Actinobacillus pleuropneumoniae (A. pleuropneumoniae) in Young Pigs. PlosOne, http://dx.doi.org/10.1371/journal.pone.0161832
Zhang, H., T. Xu, M. Elhoseiny, X. Huang, S. Zhang, A. Elgammal, D. Metaxa. 2016. SPDA-CNN: Unifying Semantic Part Detection and Abstraction for Fine-grained Recognition. http://dx.doi.org/10.1109/CVPR.2016.129
All the information gathered by the system can be presented to the farmer on, for instance, a mobile device. Important is that the information is useful for the farmer and we will therefore develop the methods and visualization of the data in close collaboration with the end users of the system.